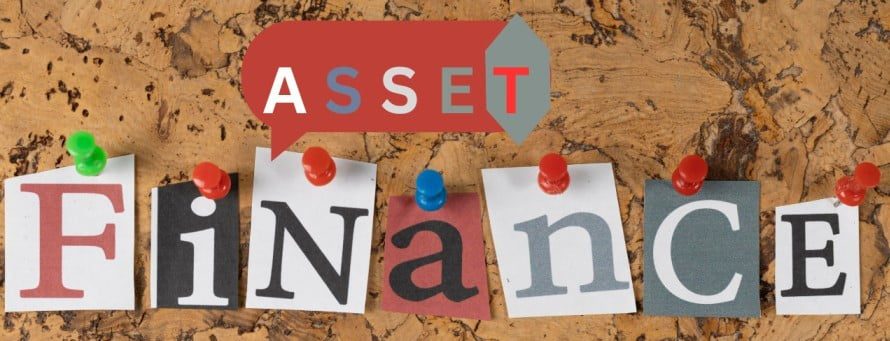
Table of Contents
Transforming Asset Finance with Machine Learning: Unlocking the Power of Data-Driven Insights
The asset finance sector is undergoing major changes as machine learning (ML) and artificial intelligence (AI) are being integrated. Financial institutions are using ML to improve operations, customer experience, and gain a competitive advantage as the need for personalized data-driven solutions grows.
The Role of Machine Learning in Asset Finance
The asset finance sector can be revolutionized by machine learning algorithms, which can analyze large amounts of data, reveal concealed patterns, and produce predictive insights. Financial institutions are being transformed in their approach to decision-making by ML, from credit scoring and fraud detection to investment management and personalized finance.
Credit Scoring and Risk Management of Asset Finance with Machine Learning
Analyzing historical data, machine learning models like decision trees and support vector machines can create more precise and reliable credit scoring systems. This allows financial institutions to evaluate customer creditworthiness with greater effectiveness, resulting in enhanced risk management and better portfolio performance. This shows the potential mechanism of asset finance with machine learning in the domain of credit scoring and risk management.
Fraud Detection and Security Monitoring
ML-driven algorithms have the capability to identify irregularities and pinpoint questionable behavior as it happens, allowing financial institutions to take proactive measures to reduce the risk of fraud and improve security monitoring. This doesn’t just safeguard the assets of the institution but also fosters increased trust among their customer base.
Investment Management and Algorithmic Trading
Machine learning has completely transformed how financial institutions handle asset allocation and portfolio optimization. By integrating ML algorithms to examine market data, recognize patterns, and make well-informed trading decisions, financial institutions are able to create more successful and profitable investment strategies.
Personalized Finance and Customer Engagement
Machine learning models can leverage customer data to provide personalized financial recommendations, tailored products, and enhanced customer experiences. This personalization can lead to increased customer satisfaction, loyalty, and ultimately will bring better financial outcomes for both the customer and the institution.
Thruster Finance: A Case Study in ML-Powered Asset Finance
Thruster Finance, a leading fintech company, has been at the forefront of leveraging machine learning in the asset finance industry. By developing a robust SaaS platform, Thruster Finance has empowered financial institutions to harness the power of data-driven insights and enhance their asset finance operations.
The Thruster Finance platform leverages advanced ML algorithms, including deep learning and natural language processing (NLP), to streamline various aspects of asset finance, such as demand forecasting, underwriting processes, and personalized customer experiences. The platform’s DevOps knowledge and CI/CD capabilities guarantee smooth and efficient product development, enabling financial institutions to remain ahead of the competition.
Thruster Finance’s platform has significantly benefited its clients by empowering them to make better decisions, streamline asset portfolios, and improve customer interaction. By harnessing the power of machine learning, Thruster Finance has helped its clients navigate the complex financial landscape and achieve greater success in the asset finance domain. Indeed, such platform has shown the pivotal integrity of asset finance with machine learning.
The Future of Asset Finance: Embracing the ML Revolution
The financial sector is evolving, making the use of machine learning and artificial intelligence more important for asset finance institutions. Some of the key trends and developments that will shape the future of this dynamic sector:
- Adhering to regulations and ethical standards: Financial institutions will need to ensure that their ML-powered systems adhere to strict regulatory guidelines and ethical principles, maintaining transparency and accountability in their decision-making processes.
- Democratization of Financial Services: Machine learning will play a vital role in making financial services more accessible and inclusive. It will empower individuals and small businesses to make informed financial decisions.
- Predictive Analytics and Demand Forecasting: Advanced ML algorithms will enable financial institutions to accurately forecast demand, optimize inventory management, and streamline their asset finance operations.
- Intelligent Automation and Intelligent Assistants: The integration of robotic process automation (RPA) and natural language processing (NLP) will automate repetitive tasks, freeing up human resources to focus on more strategic initiatives. Intelligent chatbots and virtual assistants will enhance customer engagement and provide personalized support.
- Ecosystem Integration and the Internet of Things: Machine learning will have a crucial role in enabling smooth ecosystem integration and uncovering new opportunities in asset finance as the financial industry merges with other sectors like logistics, e-commerce, and e-gaming.
Conclusion
Machine learning integration in the asset finance sector is reshaping how financial institutions handle decision-making, customer interaction, and portfolio enhancement. Leveraging data-driven insights enables these institutions to improve their competitive advantage, streamline operations, and offer enhanced financial services.
As the financial industry progresses, the incorporation of machine learning and artificial intelligence will be crucial for the prosperity and durability of asset finance firms. Embracing this technology-driven shift allows financial institutions to explore fresh opportunities, foster innovation, and fortify their standing in the swiftly evolving financial environment. In short, financial institutions are more reliable and compatible in terms of transforming asset finance with machine learning tools.
For more latest blog post on Business: